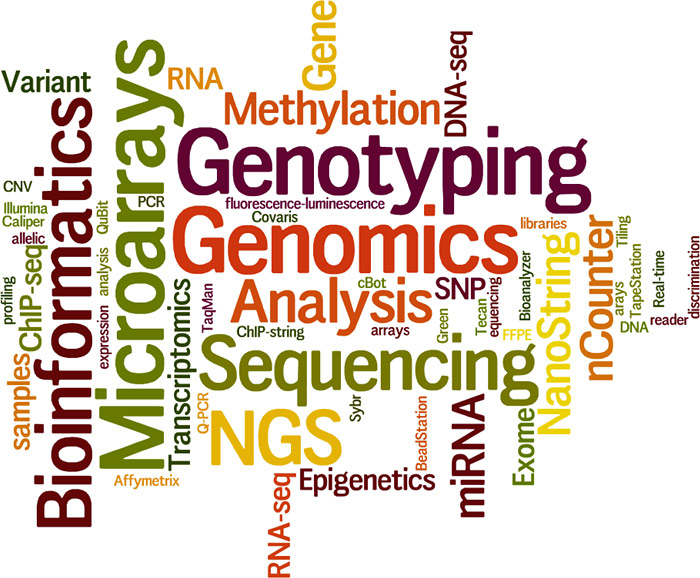
High-throughput Genomics
The information required to maintain and reproduce life is encoded in our DNA and organized in genes. The entirety of an organism’s genes (thus its hereditary information) is called a genome. Genomes, control the function (or dysfunction) of organisms according to their “expression” through an essential process called the “central dogma of molecular biology”, an explanation of the flow of genetic information within a biological system leading to proteins, final products responsible for a variety of crucial biological functions. Genomics is a scientific area that concerns the sequencing and analysis of an organism’s genome. Experts in genomics strive to determine complete DNA sequences and perform genetic mapping to help understand disease.
The real revolution in genomics arrived with the introduction of the massively parallel sequencing platforms which represent a new generation of DNA sequencing called Next Generation Sequencing (NGS) and which produces terabytes of sequencing data in short times with continuously decreasing cost. The NGS technology has already proved to have tremendously more applications than originally anticipated. Some of them include the de novo genome sequencing or re-sequencing or the study of chromatin methylations and genome wide protein-DNA interactions, indicating potential drug targets for personalized medicine approaches. Additionally, NGS platforms are gradually replacing genomic methods, such as microarray-based analysis of gene expression, because the data generated in a single run offer wider dynamic range of measurements and additional genomic information. Other applications include the detection of genomic and genome structure variations related to genetic/inherited diseases or cancer. In addition, as NGS applications are maturing and the costs are continuously dropping, the technology is gradually evolving from its presence to the biological lab to a valuable diagnostic tool in personalized medicine as well as a generic prognostic tool which can improve overall healthcare and well-being.
Currently, the meaningful analysis and interpretation of high throughput molecular data comprises the main bottleneck in their exploitation towards the design of novel therapeutic and diagnostic strategies as well as healthcare prognostics in everyday life. The following describe HybridStat’s approaches for the analysis of various types of genomics data.
RNA-Seq data analysis
Description
RNA sequencing (RNA-Seq) is a modern approach to transcriptome profiling that uses deep-sequencing NGS technologies and has revolutionized the exploration of gene expression. Studies using this method have already altered the view of the extent and complexity of eukaryotic transcriptomes. Advances in the RNA sequencing workflow, from sample preparation through data analysis, enable rapid profiling and deep investigation of the transcriptome (totality of genes that code for proteins). Next-generation RNA sequencing enables researchers to:
- Identify and quantify both rare and common transcripts, with over six orders of magnitude of dynamic range
- Align sequencing reads across splice junctions, and detect isoforms, novel transcripts and gene fusions
- Perform robust whole-transcriptome analysis on a wide range of samples, including possibly low-quality samples as the technique is very sensitive and as the technology advances, lower quantities of initial biomaterial is required.
- Obtain high-quality and noise free results from low quantities of input material, as the technique is inherently robust to noise (e.g. there are no cross-hybridization issues like in microarrays).
In a clinical setting, RNA-Seq technology can be used in a variety of contexts such as:
- Identification of diagnostic or prognostic biomarkers based on gene expression signatures under disease states
- Classification of diseases based on genetic signatures which are indistinguishable by less sensitive techniques (e.g. microscopy) and are results of processes difficult to quantitate (e.g. alternative splicing and differential isoform expression).
- Understand complex and sensitive mechanisms involved in the genesis of disease processes
create comprehensive and detailed gene expression maps in several tissues and disease states in order to catalog possible drug targets for personalized gene therapies
HybridStat offers RNA-Seq data preprocessing including short sequence mapping to reference genomes, data quality checks and filtering, normalization, statistical analysis, data visualization and more for all major technology platforms (Illumina, SOLiD, Ion Torrent). In addition, HybridStat offers consulting regarding the experimental design for optimal downstream statistical analysis.
Output
Provided with the raw short sequence reads, HybridStat generates friendly reports with:
- Comprehensive, detailed and annotated (through the usage of related biological databases) gene lists coupled with statistical significance and several confidence metrics for the followed experimental design
- Lists of biochemical pathways and biological functions where these genes are involved, coupled with statistical significance and several confidence metrics
- Friendly and established data visualization of the results (gene expression heatmaps, volcano plots)
- Friendly and established quality diagnostics of the raw data (alignment statistics, sequenced reads qualities) as well the downstream data analysis (boxplots, mean difference plots etc.)
- Genome browser visualizations for visual inspection of sequencing results and correlation with other annotations
- Much more RNA-Seq analytics which can be derived upon discussions with the client
ChIP-Seq data analysis
Description
Transcription factors (TFs) are DNA-associated proteins which are essential in genotype-phenotype mapping. Determining DNA interaction and regulation mechanisms is crucial for unraveling the complexity of many biological processes and disease states. This epigenetic information is complimentary to genotypic and gene expression analysis. Several studies suggest that perturbations in transcriptional networks can promote several diseases including cancer. For example, TF Prox1 has been shown to induce colon cancer progression by promoting phenotype transition from benign to dysplastic and the activity of Cdx2 limits the proliferation of human colon cancer cells by inhibiting the transcriptional activity of the β-catenin – T-cell factor (TCF) bipartite complex. Besides TF activities, other important epigenetic factors of systematic perturbations guiding disease states in cancers are DNA methylations.
ChIP-sequencing, also known as ChIP-Seq, is a method used to analyze protein interactions with DNA. ChIP-seq combines chromatin immunoprecipitation (ChIP) with massively parallel DNA sequencing to identify the binding sites of DNA-associated proteins. It can be used to map global binding sites precisely for any protein of interest as well as the study of chromatin methylations. The goal of ChIP-Seq data analyses is to find enriched genomic regions (peaks) in a pool of precipitated DNA fragments and the output of peak-finding methods is usually a list of ‘peak calls’ comprising the genomic locations of sites inferred to be occupied by the protein.
HybridStat offers ChIP-Seq data preprocessing including short sequence mapping to reference genomes, data quality checks and filtering, normalization, enriched region calling and association with closest genomic features of interest – targets, statistical analysis, data visualization and more for all major technology platforms (Illumina, SOLiD, Ion Torrent). In addition, HybridStat offers consulting regarding the experimental design for optimal downstream statistical and biological analysis.
Outcome
Provided with the raw short sequence reads, HybridStat generates friendly reports with:
Comprehensive, detailed and quality checked (bioinformatically) signal enriched DNA regions (ChIP-Seq peaks or enriched methylated regions) coupled with statistical significance and several confidence and quality metrics
- Annotation of the enriched regions with their closest genomic features (e.g. genes) with which they might be functionally interacting
- Friendly and established data analytics and visualization of the results (genomic distribution of the enriched DNA binding signals, signal profiles across genomic features of interest and more)
- Friendly and established quality diagnostics of the raw data (alignment statistics, sequenced reads qualities) as well the downstream data analysis (signal-to-noise ratio plots, signal saturation etc.)
- Genome browser visualizations for visual inspection of sequencing results and correlation with other annotations
- Much more ChIP-Seq analytics which can be derived upon discussions with the client
Whole Exome Sequencing data analysis
Description
DNA variants (variations from a representative, reference consensus genome) are one (Single Nucleotide Polymorphisms –SNPs) or more DNA nucleotides that are responsible for altering the protein product structure, rendering in non-functional or deleterious. When the variant consists of more than one DNA nucleotide, then this group of nucleotides may be deleted from the reference as a result of a genetic disease (DNA deletion) or be multiplied (DNA insertions). The exome represents less than 2% of the human genome, but contains ~85% of known disease-causing DNA variants, making whole-exome sequencing (Exome-Seq) a cost-effective alternative to whole-genome sequencing. On the other hand, as DNA variants can occur outside known protein-coding regions (for example in gene promoter regions which have strong functional characteristics for the functional integrity of an organism), the detection of DNA mutations in such regions is also importance. The latter can be achieved with whole genome or DNA sequencing (DNA-Seq).
Exome sequencing is a technique for sequencing all the protein-coding genes in a genome (known as the exome). It consists of first selecting only the subset of DNA that encodes proteins (known as exons), and then sequencing that DNA using any high throughput DNA sequencing technology. There are 180,000 exons, which constitute about 1% of the human genome, or approximately 30 million base pairs, but mutations in these sequences are much more likely to have severe consequences than in the remaining 99%.With exome sequencing, researchers can investigate the protein coding regions of the genome when sequencing an entire genome is not practical or necessary. It can efficiently identify variants across a wide range of applications, including population genetics, genetic disease, and cancer studies. Exome sequencing is especially effective in the study of rare Mendelian diseases, because it is the most efficient way to identify the genetic variants in all of an individual’s genes and is one of the most promising technologies to reach the goal of personalized diagnosis and medicine.
Output
Provided with the raw short sequence reads, HybridStat generates friendly reports with:
- Comprehensive, detailed and annotated (through the usage of related biological databases and reference genome annotations) DNA alteration lists coupled with their exact locations, statistical significance and several confidence metrics.
- Lists of biochemical pathways and biological functions where the genes with detected DNA alterations are involved, with statistical significance and several confidence metrics
- Friendly and established data visualization of the results (chromosomal localizations, involved pathways)
- Friendly and established quality diagnostics of the raw data as well the data analysis
- Genome browser visualizations for visual inspection of sequencing results and correlation with other annotations
- Much more analytics which can be derived upon discussions with the client
DNA microarray data analysis
Description
DNA microarray analysis is an established high-throughput technology for monitoring whole genomes in the field of genetic research. Scientists are using DNA microarrays to investigate everything from cancer to pest control. For example, in the case of disease study, microarrays are used by researchers and biotechnology companies to:
- Identify diagnostic or prognostic biomarkers
- Classify diseases (e.g. tumors with different prognosis that are indistinguishable by microscopic examination)
- Monitor the response to therapy
- Understand the mechanisms involved in the genesis of disease processes
- Catalog potential drug targets of genetic therapies for a variety of diseases
DNA microarray data analysis requires specialized statistical expertise and bioinformatics knowledge to convert raw signals from laser scanned images to biologically meaningful measurements and identify gene biomarker candidates. HybridStat offers microarray data preprocessing, normalization and statistical analysis of gene expression profiling studies for all the major commercial microarray providers (Affymetrix, Illumina, Agilent) as well as custom microarray platforms and exon arrays. Analysis based on several widely used open-source tools as well as proprietary in-house workflows. HybridStat also offers assistance in the experimental design for optimal downstream statistical analysis.
Output
Provided with the raw scanned microarray image signals, HybridStat generates friendly reports with:
- Comprehensive, detailed and annotated (through the usage of related biological databases) gene lists coupled with statistical significance and several confidence metrics for the followed experimental design
- Lists of biochemical pathways and biological functions where these genes are involved, coupled with statistical significance and several confidence metrics
- Friendly and established data visualization of the results (gene expression heatmaps, volcano plots)
- Friendly and established quality diagnostics of the raw data as well the data analysis (boxplots, mean difference plots etc.)